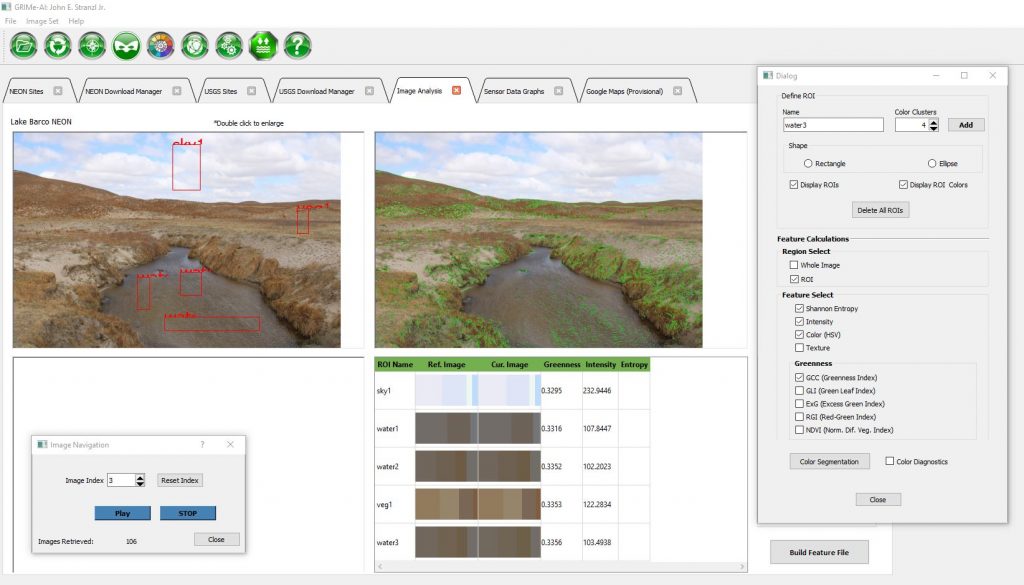
This post builds on our recent update about GRIME-AI capabilities. The previous post (and video) described features in GRIME-AI that are reasonably stable (although subject to occasional changes in APIs for public data sources). The description below is our current roadmap to a full GRIME-AI suite of tools for using imagery in ecohydrological studies. Please contact us if you see major gaps or are interested in helping us test the software as new features are developed!
The following features are implemented or planned for GRIME-AI:
You will notice asterisks that indicate *planned future functionality (timeframe = months to years) and **functionality under development (timeframe = weeks to months). All other features are developed, but subject to additional user testing as we work toward a stable public release. GRIME-AI is being developed open source commercial friendly (Apache 2.0).
- Acquire PhenoCam imagery and paired NEON sensor data
- Acquire USGS HIVIS imagery and paired stage, discharge and other sensor data
- Data cleaning (image triage)
- Automatically identify and remove low-information imagery
- Data fusion*
- Identify gaps in image and other sensor data*
- Documented resolution of data gaps*
- Drop imagery or other sensor data where all data is not available, select interpolation method to fill small gaps, and/or train/test ML models that use imagery to fill other sensor data gaps*
- Documented data alignment criteria*
- Choose precision for “paired” timestamps (e.g., +/- 5 min between image timestamp and other sensor data timestamp)*
- Image analysis
- Calculate and export scalar features for ML with low computational requirements
- Image analysis algorithms include:
- K-means color clustering (user selected, up to 8 clusters, HSV for each cluster)
- Greenness index (PhenoCam approach)
- Shannon Entropy
- Intensity
- Texture
- Image analysis algorithms include:
- Draw masks for training segmentation models**
- Draw polygon shapes
- Save masks and overlay images**
- Export mask**
- Image calibration and deterministic water level detection (currently a separate Windows installer called GRIME2, but we have command line to implement this in GRIME-AI)**
- Draw calibration ROI for automatic detection of octagon calibration targets
- Draw edge detection ROI for automatic detection of water edge
- Enter reference water level and octagon facet length
- Process image folders
- Save overlay images
- All scalar feature values, ROIs and polygon shapes exported as .csv and .json*
- Calculate and export scalar features for ML with low computational requirements
- Data products and export*
- Documentation of data source and user decisions, where final datasets include:
- Metadata for all source data*
- Documented user decisions from data cleaning and data fusion processes*
- Documentation of calculated image features*
- Sample image overlay showing location of ROIs*
- Sample image showing segmentation masks and labels*
- Coordinates and labels of all ROIs (.csv and .json)*
- Breakpoints for major camera movement, image stabilization, or other major changes in imagery*
- A .csv and a .json file with aligned, tidy data that is appropriate for training/testing ML models*
- Metadata appropriate for storing final data product (scalar data only) in CUAHSI HydroShare or similar data repository*
- Documentation of imagery source, including timestamps and metadata for original imagery retrieved from public archive*
- Documentation of data source and user decisions, where final datasets include:
- Modeling and model outputs*
- Build segmentation models (e.g., to automatically detect water surfaces in images)*
- Build ML models from scalar image features and other sensor data and/or segmentation results*
- Export model results, performance metrics, and metadata*
All of the above are being developed under the MESH development philosophy:
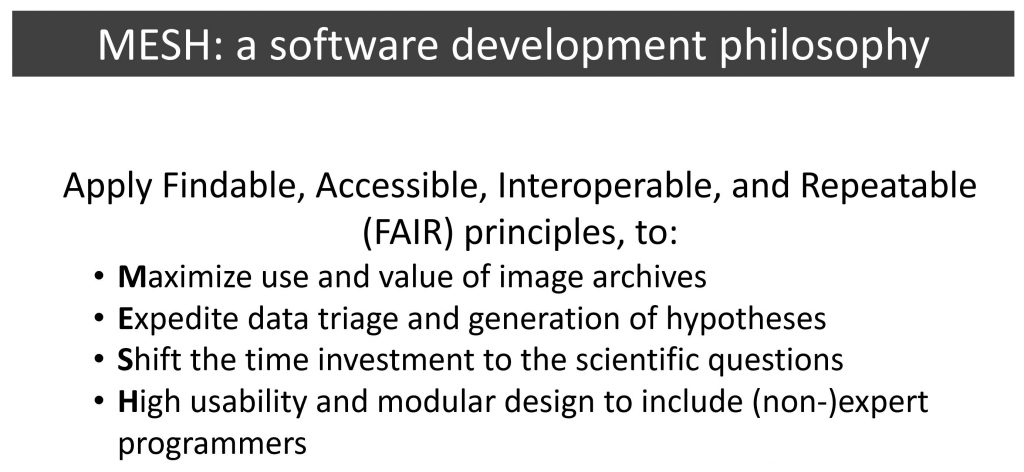